Likewise, I'm glad to hear there was some confirmation from your team!
An option for you if you don't want to do a full writeup is to make a "diff" or comparison post, just listing where your methods and results were different (or the same). I think there's demnad for that, people liked Comparing Anthropic's Dictionary Learning to Ours
I've had a lot of conversations with people lately about OthelloGPT and I think it's been useful for creating consensus about what we expect sparse autoencoders to recover in language models.
I'm surprised how many people have turned up trying to do something like this!
What is the performance of the model when the SAE output is used in place of the activations?
I didn't test this.
What is the L0? You say 12% of features active so I assume that means 122 features are active.
That's correct. I was satisfied with 122 because if the SAEs "worked perfectly" (and in the assumed ontology etc) they'd decompose the activations into 64 features for [position X is empty/own/enemy], plus presumably other features. So that level of density was acceptable to me because it would allow the desired ontology to emerge. Worth trying other densities though!
In particular, can you point to predictions (maybe in the early game) where your model is effectively perfect and where it is also perfect with the SAE output in place of the activations at some layer? I think this is important to quantify as I don't think we have a good understanding of the relationship between explained variance of the SAE and model performance and so it's not clear what counts as a "good enough" SAE.
I did not test this either.
At a high level, you don't get to pick the ontology.
I agree, but that's part of what's interesting to me here - what if OthelloGPT has a copy of a human-understandable ontology, and also an alien ontology, and sparse autoencoders find a lot of features in OthelloGPT that are interpretable but miss the human-understandable ontology? Now what if all of that happens in an AGI we're trying to interpret? I'm trying to prove by example that "human-understandable ontology exists" and "SAEs find interpretable features" fail to imply "SAEs find the human-understandable ontology". (But if I'm wrong and there's a magic ingredient to make the SAE find the human-understandable ontology, lets find it and use it going forward!)
Separately, it's clear that sparse autoencoders should be biased toward local codes over semi-local / compositional codes due to the L1 sparsity penalty on activations. This means that even if we were sure that the model represented information in a particular way, it seems likely the SAE would create representations for variables like (A and B) and (A and B') in place of A even if the model represents A. However, the exciting thing about this intuition is it makes a very testable prediction about combinations of features likely combining to be effective classifiers over the board state. I'd be very excited to see an attempt to train neuron-in-a-haystack style sparse probes over SAE features in OthelloGPT for this reason.
I think that's a plausible failure mode, and someone should definitely test for it!
I found your bolded claims in the introduction jarring. In particular "This demonstrates that current techniques for sparse autoencoders may fail to find a large majority of the interesting, interpretable features in a language model".
I think our readings of that sentence are slightly different, where I wrote it with more emphasis on "may" than you took it. I really only mean this as an n=1 demonstration. But at the same time, if it turns out you need to untie your weights, or investigate one layer in particular, or some other small-but-important detail, that's important to know about!
Moreover, I think it would best to hold-off on proposing solutions here
I believe I do? The only call I intended to make was "We hope that these results will inspire more work to improve the architecture or training methods of sparse autoencoders to address this shortcoming." Personally I feel like SAEs have a ton of promise, but also could benefit from a battery of experimentation to figure out exactly what works best. I hope no one will read this post as saying "we need to throw out SAEs and start over".
Negative: I'm quite concerned that tieing the encoder / decoder weights and not having a decoder output bias results in worse SAEs.
That's plausible. I'll launch a training run of an untied SAE and hopefully will have results back later today!
Oh, and maybe you saw this already but an academic group put out this related work: https://arxiv.org/abs/2402.12201
I haven't seen this before! I'll check it out!
[Continuing our conversation from messages]
I just finished a training run of SAEs on the intermediate layer of my OthelloGPT. For me it seemed like the sweet spot was layers 2-3, and the SAE found up to 30 high-accuracy classifiers on Layer 3. They were located all in the "inner ring" and "outer ring", with only one in the "middle ring". (As before, I'm counting "high-accuracy" as AUROC>.9, which is an imperfect metric and threshold.)
Here were the full results. The numbers/colors indicate how many classes had a high-accuracy classifier for that position.
Good thinking, here's that graph! I also annotated it to show where the alpha value I ended up using for the experiment. Its improved over the pareto frontier shown on the graph, and I believe thats because the data in this sweep was from training for 1 epoch, and the real run I used for the SAE was 4 epochs.
Nope. I think they wouldn't make much difference - at the sparsity loss coefficient I was using, I had ~0% dead neurons (and iirc the ghost gradients only kick in if you've been dead for a while). However, it is on the list of things to try to see if it changes the results.
Cool! Do you know if they've written up results anywhere?
Here are the datasets, OthelloGPT model ("trained_model_full.pkl"), autoencoders (saes/), probes, and a lot of the cached results (it takes a while to compute AUROC for all position/feature pairs, so I found it easier to save those): https://drive.google.com/drive/folders/1CSzsq_mlNqRwwXNN50UOcK8sfbpU74MV
You should download all of these into the same level directory as the main repo.
Thanks for doing this -- could you share your code?
Just uploaded the code here: https://github.com/RobertHuben/othellogpt_sparse_autoencoders/. Apologies in advance, the code is kinda a mess since I've been writing for myself. I'll take the hour or so to add info to the readme about the files and how to replicate my experiments.
I'd be interested in running the code on the model used by Li et al, which he's hosted on Google drive:
https://drive.google.com/drive/folders/1bpnwJnccpr9W-N_hzXSm59hT7Lij4HxZ
Thanks for the link! I think replicating the experiment with Li et al's model is definite next step! Perhaps we can have a friendly competition to see who writes it up first :)
I have mixed feelings about whether the results will be different with the high-accuracy model from Li et al:
Also, in addition to the future work you list, I'd be interested in running the SAEs with much larger Rs and with alternative hyperparameter selection criteria.
Agree that its worth experimenting with R, but the only other hyperparameter is the sparsity coefficient alpha, and I found that alpha had to be in a narrow range or the training would collapse to "all variance is unexplained" or "no active features". (Maybe you mean Adam hyperparameters, which I suppose might also be worth experimenting with.) Here's the result of my hyperparameter sweep for alpha:
In my accounting, the word "arbitrarily" saved me here. I do think I missed the middle ground of the sandboxed, limited programming environments like you.com and the current version of ChatGPT!
Followup on tied vs untied weights: it looks like untied makes a small improvement over tied, primarily in layers 2-4 which already have the most classifiers. Still missing the middle ring features though.
Next steps are using the Li et al model and training the SAE on more data.
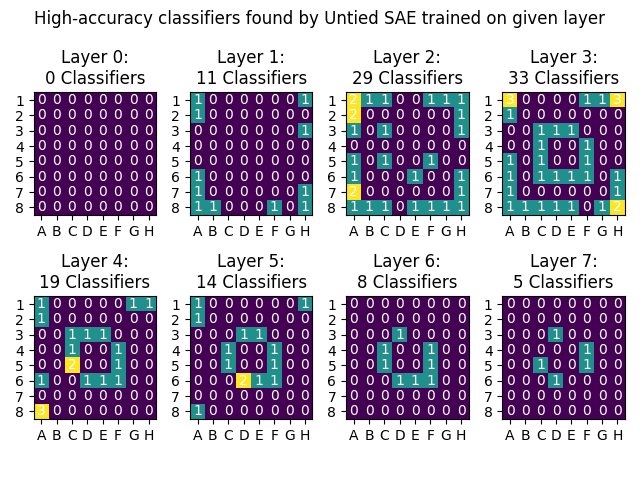
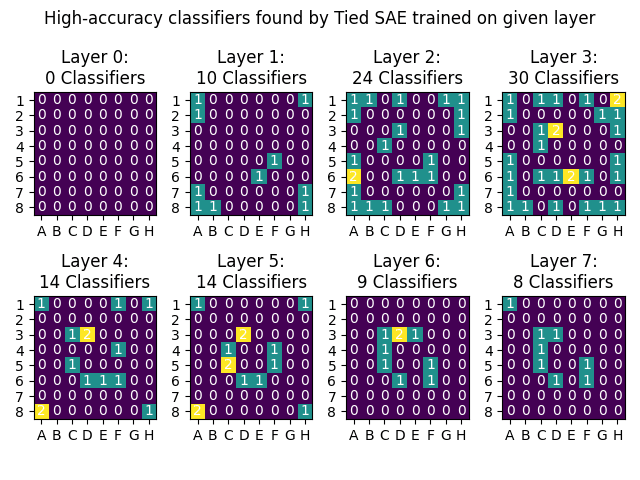