Several submissions contained perspectives, tricks, or counterexamples that were new to us. We were quite happy to see so many people engaging with ELK, and we were surprised by the number and quality of submissions.
A thing I'm curious about: what's your 'current overall view' on ELK? Is this:
From my perspective, ELK is currently very much "A problem we don't know how to solve, where we think rapid progress is being made (as we're still building out the example-counterexample graph, and are optimistic that we'll find an example without counterexamples)" There's some question of what "rapid" means, but I think we're on track for what we wrote in the ELK doc: "we're optimistic that within a year we will have made significant progress either towards a solution or towards a clear sense of why the problem is hard."
We've spent ~9 months on the problem so far, so it feels like we've mostly ruled out it being an easy problem that can be solved with a "simple trick", but it very much doesn't feel like we've hit on anything like a core obstruction. I think we still have multiple threads that are still live and that we're still learning things about the problem as we try to pull on those threads.
I'm still pretty interested in aiming for a solution to the entire problem (in the worst case), which I currently think is still plausible (maybe 1/3rd chance?). I don't think we're likely to relax the problem until we find a counterexample that seems like a fundamental reason why the original problem wasn't possible. Another way of saying this is that we're working on ELK because of a set of core intuitions about why it ought to be possible and we'll probably keep working on it until those core intuitions have been shown to be flawed (or we've been chugging away for a long time without any tangible progress).
I don't think we're likely to relax the problem until we find a counterexample that seems like a fundamental reason why the original problem wasn't possible.
I continue to think that this is a mistake that locks out the most promising directions for solving it. It's a well-known constraint that models are generally underdetermined, so you need some sort of structural solution to this underdetermination, which you can't have if it must work for all models.
Bravo! Honestly the thing I'm most impressed with here is your blazing speed.
I like the "make it useful to another AI" idea, in part because I think it has interesting failure modes. The dynamic between the predictor and the user is apparently adversarial (so you might imagine that training the predictor on a fixed user will lead to the user getting deceived, while training the user on a fixed predictor leads to deceptions being uncovered). But also, there's a cooperative dynamic where given a fixed evaluation function for how well the user does, both the predictor and the user are trying to find exploits in the evaluator.
There is still one proposal for which I don’t think there is a known counterexample, my fifth one. Since it got an honorable mention, if I understand correctly it got classified under “reward reporters that are sensitive to what’s actually happening in the world”, with the counterexample “reporter randomizes its behavior”. I don’t think that’s right, or if it is, it might be easy to modify it so that it keeps working.
The basic idea was: Train a bayesian network or some similar model to map from [question,answer] pairs to the latent variables and activations (including observations) of the predictor. Penalize computation time. In order to answer questions, set any reasonable prior over answers and then perform bayesian inference.
Here both the “direct generator” and the “generator of worlds that a human thinks are consistent with a QA pair” (what I think you think is the counterexample) need to perform a lot of computation that scales with the size of the predictor. But assuming that we have a clean dataset where the answers correspond to reality, given an input like “What is on top of the pedestal? A diamond” the direct generator can just generate a representation of a diamond, while the other one needs to perform extra computation to determine in what other worlds a human would give that reply. This extra computation will be penalized, so the direct generator will be preferred and then the algorithm uses it to answer questions.
This was classified under "Train a reporter that's useful for an auxiliary AI."
(I think you didn't get listed under that category because you got another prize for a different proposal and we were inconsistent about listing people multiple times.)
Sorry for writing so many comments, but I just don’t see it. Unless it is implemented in a very weird way or I’m misunderstanding something, my proposal doesn’t fail to the steganography counterexamples.
I don’t know why you think it would. One possibility is that there is a superficially similar proposal that might fail to steganography: we can train a reporter so that it produces answers that allow another system to reconstruct the activations of the predictor. In this case steganography is useful to pass extra information to that generator. But my proposal isn’t trying to make the answers as informative about the world as possible (during training); if we are using a proper distribution matching generator (i.e. almost anything except GANs), then it is trying to model the distribution as accurately as possible (given the computational limitations). Encoding information on the far side of the moon would just increase its loss function: given any random variable the distribution that minimizes the expected -log-likelihood is its true distribution, not any smart encoding. And if there is a QA pair that a human could have answered with uncertainty, the generator should then again accurately model that distribution (but a well made dataset won’t reflect that uncertainty).
And even if it did perform steganography (for some reason unknown to me) it would do so “on top” of the “direct generator” instead of the “generator of worlds that a human thinks are consistent with a QA pair”, because it is simpler.
I may have misunderstood your proposal. (And I definitely misremembered it when I replied to your comment earlier, I just looked back at the google doc now.)
Here was the naive interpretation I had of what you submitted:
I think you suggested giving F many (question, answer) pairs but it wasn't clear how to define the function and I don't think it change the bottom line.
It seems like this would learn the bad behavior:
This is much cheaper than a direct translator if the human model is much cheaper than a direct translator. It leads to great log loss, and it copies human answers.
If you had something else in mind then we may have misinterpreted. I'd be happy to provide feedback if you provide pseudocode or another precise description.
Is this precise enough?
This is given by P(A|Q,z) = P(Q,A,z)/P(Q,z) = P(Q)*P(A|Q)*P(z|Q,A)/P(Q,z) ∝ P(A|Q)*P(z|Q,A)
As discussed in the document, generating the sensory data first is a problem that won’t happen. Neither can it fill the activations at random, that will give it a terrible loss.
Is this precise enough?
It seems like there are still some details that lead to totally different behavior: how do we represent the generative model, how do we define C, and maybe (depending on the answers to those questions) how do we trade off C against the log loss and how do we incorporate many different questions and answers.
In order to respond, I'll just assume that you are considering only a single question-answer pair (since otherwise there are lots of other details) and just make some random assumptions about the rest. I expect you aren't happy with this version, in which case you can supply your own version of those details.
Learn a conditional probability distribution F: (question, answer) --> (predictor activations)
- It must be such that we can compute P(predictor activations|question, answer), or some approximation
How do you represent that probability distribution?
I don't think this works, but just want to understand the proposal.
The concrete generative model I had in mind was the one I used as an example in the document (page 1 under section “Simplest implementation”):
Train a conditional VAE to generate all the variables of the decoder of the predictor, condition on the [question, answer] pair. Use L2 regularization on the decoder of our model as a proxy for complexity (since the computation time of VAEs is constant).
An autoregressive model is probably the single worst model you could choose. It forces an order of generation of the latents, which breaks the arguments I wrote in my proposal. And for the purposes of how I expect the intended model to work (which I will explain below), it is very very deep. During training we can generate everything in parallel, but at inference time we need to generate each token after the previous one, which is a very deep computational graph.
I don’t understand what you mean by considering a single question-answer pair. If for a given scenario we have multiple questions and answers, we just feed each [question, answer, activations] triple into our model as training data.
Does that sound right?
It does, that is the distribution it is modeling.
The intended behavior is the one I wrote in my reply to Thomas:
both the “direct generator” and the “generator of worlds that a human thinks are consistent with a QA pair” need to generate the activations that represent the world, but the “direct generator” does it directly (e.g. “diamond” -> representation of a diamond), while the other one performs additional computation to determine what worlds a human thinks are consistent with a QA pair (e.g. “diamond” -> it might be a diamond, but it might also be just a screen in front of the camera and what is really in the vault is…), in the training set where the labels are always correct the direct generator is preferred.
If we have a dataset where what is seen on screen always corresponds (simply) with reality, I expect the model to generate the “world” first (using a direct map like I said above) and then use it to generate the images (I argued for this order in the document). If we use this to answer questions, it will care about what is really happening in reality (this is just the Markov property, see the last row of my drawing if that isn’t clear).
If for some reason this fails and the model generates both the images and the world each conditioned mostly independently on the QA pair, then using that to answer questions will get us a system that cares about both what is happening on screen and what is happening in reality. Given that the data it had access to is consistent with both hypotheses, I don’t fault it for doing that. Using it to optimize the world so that the answer to “Is the diamond in the vault?” is “Yes”, will give us a system that cares about “both” diamonds. Not a terrible failure mode.
If on the other hand we have a dataset where the vault has an operator, so that it is hard for another person to answer correctly, the ordering argument becomes stronger. For simple enough models QA->world->images works but in QA->images->world the QA information is lost in the first step and doesn’t get to the world (since the images are modeled as independent from the true answer). And in this case if this fails for some reason and the model generates the world and the images independently, using it to answer questions will give us a model that cares strongly about reality. Because the correlation of the QA pair is stronger with reality (by construction, at least for simple models) than with the images. Here you can’t use the argument of “but what if performing perfect inference on the human simulation is still cheaper than the direct reporter”, since both models need to be big enough to generate all the data.
If you can think of another algorithm as simple as a direct generator that performs well in the training set, describe it.
Even if you think I'm wrong, do you at least understand why I currently think this might work?
The concrete generative model I had in mind was the one I used as an example in the document (page 1 under section “Simplest implementation”):
Ah, I was looking at "submission #2" for this whole discussion and didn't even notice that submission #5 was very similar, perhaps this explains much of the confusion.
I agree that a VAE with L2 on the decoder is the most promising version of this approach.
If for a given scenario we have multiple questions and answers, we just feed each [question, answer, activations] triple into our model as training data.
I meant: do you condition on a single question-answer pair at a time, or do you condition on a long list of them? It sounds like you want to condition on a single one. This seems simplest and I'm happy to focus on it, but it's a little bit scary because the log loss reduction from conditioning on just one answer is so tiny (and so it's not clear if it's worth the model spending complexity to implement conditioning at all, since the no-conditioning model is extremely simple and gets almost exactly the same loss, though I'm happy to bracket this issue for now / assume we set hyperparameters so that it's worthwhile to integrate the QA pair).
Even if you think I'm wrong, do you at least understand why I currently think this might work?
Yeah, I see the appeal of this approach and agree that (at least right now) it seems more promising than the versions of predicting latent state that can be defeated with steganography.
If you can think of another algorithm as simple as a direct generator that performs well in the training set, describe it.
Right now I'm mostly worried about something like the counterexample to "penalize depending on downstream variables." So:
It currently seems to me like this kind of counterexample would work, but this bulleted list is not yet a formal description (and it does seem somewhat harder to counterexample than depending on downstream variables). I'll think about it a bit more.
Once it observes that covert tampering did in fact occur, it stops assuming that the human will be correct. (Since the most likely explanation is either that the human messed up, or that the model underestimated human abilities.) It seems like it won't end up assuming that both tampering occurred to show a diamond and that the diamond was actually present.
But the neat thing is that there is no advantage, either to the size of the computational graph or in predictive accuracy, to doing that. In the training set the human is always right. Regular reporters make mistakes because what is seen on camera is a non-robust feature that generalizes poorly, here we have no such problems.
But I might have misunderstood, pseudocode would be useful to check that we can’t just remove “function calls” and get a better system.
Ah, a conditional VAE! Small question: Am I the only one that reserves 'z' for the latent variables of the autoencoder? You seem to be using it as the 'predictor state' input. Or am I reading it wrong?
Now I understand your P(z|Q,A) better, as it's just the conditional generator. But, how do you get P(A|Q)? That distribution need not be the same for the human known set and the total set.
I was wondering what happens in deployment when you meet a z that's not in your P(z,Q,A) (ie very small p). Would you be sampling P(z|Q,A) forever?
You aren’t the only one, z is usually used for the latent, I just followed Paul’s notation to avoid confusion.
P(A|Q) comes just from training on the QA pairs. But I did say “set any reasonable prior over answers”, because I expect P(z|Q,A) to be orders of magnitude higher for the right answer. Like I said in another comment, an image generator (that isn’t terrible) is incredibly unlikely to generate a cat from the input “dog”, so even big changes to the prior probably won’t matter much. That being said, machine learning rests on the IID assumption, regular reporters are no exception, they also incorporate P(A|Q), it’s just that here it is explicit.
The whole point of VAEs is that the estimation of the probability of a sample is efficient (see section 2.1 here: https://arxiv.org/abs/1606.05908v1), so I don’t expect it to be a problem.
Is this precise enough?
As I read this, your proposal seems to hinge on a speed prior (or simplicity prior) over F such that F has good generalization from the simple training set to the complex world. I think you could be more precise if you'd explain how the speed prior (enforced by C) chooses direct translation over simulation. Reading your discussion, your disagreement seems to stem from a disagreement about the effect of the speed prior i.e. are translators faster or less complex than imitators?
The disagreement stems from them not understanding my proposal, I hope that now it is clear what I meant. I explained in my submission why the speed prior works, but in a nutshell it is because both the “direct generator” and the “generator of worlds that a human thinks are consistent with a QA pair” need to generate the activations that represent the world, but the “direct generator” does it directly (e.g. “diamond” -> representation of a diamond), while the other one performs additional computation to determine what worlds a human thinks are consistent with a QA pair (e.g. “diamond” -> it might be a diamond, but it might also be just a screen in front of the camera and what is really in the vault is…), in the training set where the labels are always correct the direct generator is preferred.
Hmm, why would it require additional computation? The counterexample does not need to be an exact human imitator, only a not-translator that performs well in training. In the worst case there exist multiple parts of the activations of the predictor that correlate to "diamond", so multiple 'good results' by just shifting parameters in the model.
A generative model isn’t like a regression model. If we have two variables that are strongly correlated and want to predict another variable, then we can shift the parameters to either of them and get very close to what we would get by using both. In a generative model on the other hand, we need to predict both, no value is privileged, we can’t just shift the parameters. See my reply to interstice on what I think would happen in the worst case scenario where we have failed with the implementation details and that happens. The result wouldn’t be that bad.
If you can think of another algorithm as simple as a direct generator that performs well in training, say so. I think that almost by definition the direct generator is the simplest one.
And if we make a good enough but still human level dataset (although this isn’t a requirement for my approach to work) the only robust and simple correlation that remains is the one we are interested in.
Ah, I missed that it was a generative model. If you don't mind I'd like to extend this discussion a bit. I think it's valuable (and fun).
I do still think it can go wrong. The joint distribution can shift after training by confounding factors and effect modification. And the latter is more dangerous, because for the purposes of reporting the confounder matters less (I think), but effect modification can move you outside any distribution you've seen in training. And it can be something really stupid you forgot in your training set, like the action to turn off the lights causing some sensors to work while others do not.
You might say, "ah, but the information about the diamond is the same". But I don't think that that applies here. It might be that the predictor state as a whole encodes the whereabouts of the diamond and the shift might make it unreadable.
I think that it's very likely that the real world has effect modification that is not in the training data just by the fact that the world of possibilities is infinite. When the shift occurs your P(z|Q,A) becomes small, causing us to reject everything outside the learned distribution. Which is safe, but also seems to defeat the purpose of our super smart predictor.
As an aside, I think that that property of regression models, in addition to using small networks and poor regularization might be why adversarial examples exist (see http://gradientscience.org/adv.pdf). Some features might not be robust. If we have an image of a cat and the model depends on some non robust feature to tell it apart from dogs, we might be able to use the many degrees of freedom we have available to make a cat look like a dog. On the other hand if we used something like this method we would need to find an image of a cat that is more likely to have been generated from the input “dog” than from the input “cat”, it's probably not going to happen.
Could be! Though, in my head I see it as a self centering monte carlo sampling of a distribution mimicking some other training distribution, GANs not being the only one in that group. The drawback is that you can never leave that distribution; if your training is narrow, your model is narrow.
Interesting idea, but wouldn't it run into the problem that the map F would learn both valid and invalid correlations? Like it should learn both to predict that the activations representing "diamond position" AND the activations representing "diamond shown on camera" are active. So in a situation where those activations don't match it's not clear which will be preferred. You might say that in that case the human model should be able to generate those activations using "camera was hacked" as a hypothesis, but if the hack is done in a way that the human finds incomprehensible this might not work(or put another way, the probability assigned to "diamond location neurons acting weird for some reason" might be higher than "camera hacked in undetectable way", which could be the case if the encoding of the diamond position is weird enough)
I don’t think that would happen. But imagine that somehow it does happen, the regularization is too strong and the dataset doesn’t include any examples where the camera was hacked, so our model predicts both the activations of the physical diamond and the diamond image independently, what then? Try to think about any toy model of a scenario like that, any simple enough that we can analyze exactly. The simplest is that the variable on which we are conditioning the generation is an “uniformly” distributed scalar to which we apply two linear transformations to predict two values (which are meant to stand for the two diamonds) and then add gaussian noise. Given two observed values I’m pretty sure (I didn’t actually do the math but it seems obvious) that the reconstructed initial value is a weighted average of what would be predicted by either value independently. I expect that something analogous would happen in more realistic scenarios. Is this an acceptable behavior? I think so, or at least much better than any known alternative. If we used an AI to optimize the world so that the answer to “Is the diamond in the vault?” is “Yes”, it would make sure that both the real diamond and the one in the image stay in place.
Thanks for hosting this effort! If our names are on the list — should we have received an email, or keep an eye out for the future?
Btw., a bit late but if people are interested in reading my proposal, it's here: https://docs.google.com/document/d/1kiFR7_iqvzmqtC_Bmb6jf7L1et0xVV1cCpD7GPOEle0/edit?usp=sharing
It fits into the "Strategy: train a reporter that is useful for another AI" category, and solves the counterexamples that were proposed in this post (except if I missed sth and it is actually harder to defend against the steganography example, but I think not). (It won $10000.) It also discusses some other possible counterexamples, but not extensively and I haven't found a very convincing one. (Which does not mean there is no very convincing one, and I'm also not sure if I find the method that promising in practice.)
Overall, perhaps worth reading if you are interested in the "Strategy: train a reporter that is useful for another AI" category.
Thanks for writing this up! It's great to see all of the major categories after having thought about it for awhile. Given the convergence, does this change your outlook on the problem?
Did anybody think of using a lava lamp and a clock in the view area and checking to make sure the patterns aren’t repeating in the lamp at specific times (indicating a video loop)?
Random thought: Perhaps you could carefully engineer gradient starvation in order to "avoid generalizing" and defeat the Discrete modes of prediction example. You'd only need to delay it until reflection, then the AI can solve the successor AI problem.
In general: hack our way towards getting value-preserving reflectivity before values drift from "Diamonds" -> "What's labeled as a diamond by humans". (Replacing with "Telling the truth", and "What the human thinks is true" respectively).
How about this variation on training a sequence of reporters? As before, consider a collection of successively more powerful predictors. But instead of training a sequence of reporters to the human, train a series of mappings from the activations of M_N to M_(N-1), effectively treating each activation of M_(N-1) as a "question" to be answered, then finally train a human reporter for M_1. This could then be combined with any other strategy for regularizing the reporter. There is still the risk of learning a M_(N-1) simulator at some stage of the process, but this seems like a qualitatively better situation since M_(N-1) is only a little bit less complicated than M_N. A counterexample could still arise if there were discontinuous returns to extra complexity -- perhaps there's a situation where adding more compute does very little to improve the reporter, meaning M_(N+k) is only a little bit more complex than M_(N), but then M_(N+k+1) is suddenly able to use the extra complexity and the M_(N+k) simulator becomes simpler. This could perhaps be ameliorated by using P.'s Bayesian updating idea to train the reporters.
I guess it is just my lack of understanding (? ? ?), but - as far as I think I understand it - my own submission is actually hardly different (at least in terms of how it goes around the counter-examples we knew so far) from the Train a reporter that is useful to an auxiliary AI-proposal.
My idea was to simply make the reporter useful for (or rather: a necessarily clearly and honestly communicating part of) our original smart-vault-AI (instead of any auxiliary-AI), by enforcing a structure of the overal smart-vault-AI where its predictor can only communicate what to do to its "acting-on-the-world"-part by using this reporter.
Additionally, I would have enforced that there is not just one such reporter but a randomized row of them, so as to make sure that by having several different of them basically play "chinese-whispers", they have a harder time of converging on the usage of some kind of hidden code within their human-style communication.
I assume the issue with my proposal is that the only thing I explained about why those reporters would communicate in an understandable-for-humans-way in the first place was that this would simply be enforced by only using reporters whose output consists of human concepts + in between each training-step of the chinese-whisper-game, they would also be filtered out if they stopped using human concepts as their output.
My counter-example also seems similar to me than those mentioned under Train a reporter that is useful to an auxiliary AI-proposal.:
As mentioned above, the AI might simply use our language in another way than it is actually intended to be used, by hiding codes within it etc.
I am just posting this to get some feedback on where I went wrong - or why my proposal is simply not useful, apparently.
(Link to my original submission:) https://docs.google.com/document/d/1oDpzZgUNM_NXYWY9I9zFNJg110ZPytFfN59dKFomAAQ/edit?usp=sharing
Regarding
"Strategy: train a reporter which isn’t useful for figuring out what the human will believe/Counterexample: deliberately obfuscated human simulator".
If you put the human-interpreter-blinding before the predictor instead of between the predictor and the reporter, then whether or not the blinding produces an obfuscated human simulator, we know the predictor isn't making use of human simulation.
An obfuscated human simulator would still make for a rather bad predictor.
I think this proposal might perform slightly better than a setup where we expand the training environment to situations the supervisor doesn't understand and train the controller to take no actions when the human supervisor reports that they don't understand the data. I think the behaviour you'd get in this setup is similar to what you'd get with a perfectly obfuscated human simulator, and in practice you probably wouldn't get perfectly obfuscated simulator. Maybe it could nevertheless achieve a similar level of safety, in terms of guarantees against exploiting gaps in the supervisor's knowledge. I could be wrong on either point, I've only thought about it a little.
Below is a summary of my (honorable mention) proposal, which tries to induce mind-blindness on the Reporter. I've also posted the full contest entry as a blog post here: https://calvinmccarter.wordpress.com/2022/02/19/mind-blindness-strategy-for-eliciting-latent-knowledge/ . I've not fully analyzed whether it survives previously mentioned counterexamples, or whether it has additional unique vulnerabilities compared to other strategies, so I'd welcome feedback on these questions.
The overall strategy is to avoid training a “human simulator” reporter by regularizing its internal state to have mind-blindness. One could imagine training a “Human Simulator” that takes as input the “what’s going on” state, plus a question about what a human believes about the world, and is trained to maximize its accuracy at predicting the human’s answer explanation. Meanwhile, the Reporter is trained with dual objectives: in addition to (as before) answering questions about the world correctly, it should be penalized for containing internal state that is useful to the Human Simulator. To do this, an auxiliary “Human Simulator Detector” model is trained to predict the Human Simulator’s activations from the Reporter’s activations. The Reporter is trained adversarially to minimize the Human Simulator Detector’s accuracy. The reporter would be trained not merely to be bad at predicting the explanation — an evil reporter could pretend to be bad at predicting the explanation — but to not contain state that is predictive of “human simulator state”. This is something that the various defeated strategies are missing. A devious reporter can easily hide its full knowledge from its predicted answer, and may even choose weights to look like it does not contain a human simulator. But we argue that when operating on actual input data, a devious reporter will find it more challenging to run a simulation while pretending not to.
I mentioned it in my standalone post but I'll register a question here:
In the counterexamples for 'Strategy: train a reporter that is useful for another AI', the main difficulty is the ability for agents to hide information in human language somehow, given the many available degrees of freedom.
I grant that this is a big risk but one advantage we have is that if we trained multiple agents, they would all be encoding hidden information, but most likely they would all encode this extra information in different ways.
The question is, given multiple agents encoding hidden messages in the reporter's answers, can we find a training strategy which penalizes or removes any kind of information in the answers which is not common to all agents?
My suggestion, based on my idea of internal note-taking rather than reporting per-se, would be to take the 'notes' on the internal state written by one agent and feeding these to another agent. This seems like it would eventually converge on them sharing the same hidden encoding though, albeit with more difficulty. Apologies if not clear more spelled out in the full post, but yeah, are there better proposals, or reasons why this kind of strategy wouldn't work?
If human simulation is much simpler and faster than direct translation, then an obfuscated human-simulator would also be simpler and faster than a direct translator.
That is not obvious at all, indistinguishable obfuscation is a problem that seems to inherently require enormous amounts of computation. From the Wikipedia page you linked:
There have been attempts to implement and benchmark IO candidates. For example, as of 2017, an obfuscation of the function x1∧x2∧…∧x32 at a security level of 80 bits took 23.5 minutes to produce and measured 11.6 GB, with an evaluation time of 77 ms. Additionally, an obfuscation of the Advanced Encryption Standard hash function at a security level of 128 bits would measure 18 PB and have an evaluation time of about 272 years.
And the line below that links to a paper about a method that is exponential on the size of the circuit to obfuscate:
To give an idea about the practicality of this construction, consider a 2-bit multiplication circuit. It requires 4 inputs and between 1 and 8 AND gates for each of its 4 output bits. An obfuscation would be generated in about 10**27 years on a 2,6 GHz CPU and would require 20 Zetta Bytes of memory for m = 1 and p = 1049. Executing this circuit on the same CPU would take 1.3 × 10**8 years.
I was unable to find anything better and doubt this could be improved to the point where it would be hard to distinguish from a simple direct translator.
My thinking is: if you can do IO at all, then you can do it with a constant factor blowup (depending only on the security parameter), so if the gap between human simulator and direct translator can be arbitrarily large then this only closes a constant amount of the gap. And requiring the breaker to use the full power of IO is relatively optimistic.
ETA: looking at this in a more zoomed out way, I'd say the current state of affairs is that it's often very hard to say much about "what a model is doing" given its weights, and if you specifically train it to be incomprehensible then it feels like it could quickly get much worse. You might wonder what the theoretical situation is---in the limit does the situation favor the obfuscator or the interpreter?---and IO is mostly relevant because it shows that the obfuscator will win in the limit (for the particular quantitative question that matters for evaluating these proposals). This isn't supposed to be a bizarre claim supported only by appeal to IO (although that would suffice for a counterexample in our methodology), it is intended to be consistent with the intuitive sense of despair that many people (e.g. me) get when they stare at some giant weight matrices, already find them fairly hard to make sense of, and then imagine them being optimized to be incomprehensible.
Since the point of the contest is to discover a method for which there is no conceivable way it could fail, I grant you that given the uncertainty about what would really happen that counterexample suffices. But I still believe that in practice the interpreter will win. I think you are overextending your intuition, neural networks are incomprehensible to you because you are human, but activations in a network are what they are because they have been optimized so that the rest of the network can process (“understand”) them. So if a value can be written and read by a network, another one could do the same, since they are both being optimized to do it. It is only through complex cryptographic magic that we can avoid that (and maybe not even then if it turns out that IO can only create programs that are exponentially large).
I also believe that gradient descent can learn to use the activations of an obfuscated reporter (and indeed I frequently rely on some currently-plausible intuitive assumption like "gradient descent can't obfuscate something from itself"). But this isn't enough for these proposals to work, they need the relationship between the reporter and its obfuscated version to be quantitatively "simpler" than the relationship between the direct translator and human simulator.
Congratulations to all the winners!
And, as said before: bravo to the swift processing of all the proposals! 197 proposals, wow!
Question to those who had to read all those proposals:
How about the category of proposals* that tried to identify the good from the bad reporter by hand (with some strategy) after training multiple reporters (post hoc instead of a priori)? Were there any good ones? Or can "identification by looking at it" (either in a simple or a complex way) be definitively ruled out (and as result, require all future efforts in research to be about creating a strategy that converges to a single point on the ridge of good solutions in parameter/function space)?
Edit: * to which "Use the reporter to define causal interventions on the predictor" sort of belongs
I think we'd basically declare victory if a human could look at 2 reporters (or a bunch of reporters), one of which is a direct translator, and tell which it was The action is just in "how does the human tell?" And the counterexample is "what if the human doesn't think of anything clever, so they just have to use one of the current proposals (each of which has counterexamples)."
I didn't see the proposals, but I think that almost all of the difficulty will be in how you can tell good from bad reporters by looking at them. If you have a precise enough description of how to do that, you can also use it as a regularizer. So the post hoc vs a priori thing you mention sounds more like a framing difference to me than fundamentally different categories. I'd guess that whether a proposal is promising depends mostly on how it tries to distinguish between the good and bad reporter, not whether it does so via regularization or via selection after training (since you can translate back and forth between those anyway).
(Though as a side note, I'd usually expect regularization to be much more efficient in practice, since if your training process has a bias towards the bad reporter, it might be hard to get any good reporters at all.)
If I'm mistaken, I'd be very interested to hear an example of a strategy that fundamentally only works once you have multiple trained models, rather than as a regularizer!
If you try to give feedback during training, there is a risk you'll just reward it for being deceptive. One advantage to selecting post hoc is that you can avoid incentivizing deception.
I agree with you (both). It's a framing difference iff you can translate back and forth. My thinking was that the problem might be setup so that it's "easy" to recognize but difficult to implement. If you can define a strategy which sets it up to be easy to recognize, that is.
Another way I thought about it, is that you can use your 'meta' knowledge about human imitators versus direct translators to give you a probability over all reporters. Approaching the problem not with certainty of a solution but with recognized uncertainty (I refrain from using 'known uncertainty' here, because knowing how much you don't know something is hard).
I obviously don't have a good strategy, else my name would be up above, haha.
But to give it my attempt: One way to get this knowledge is to study for example the way NNs generalize and studying how likely it is that you create a direct translator versus an imitator. The proposal I send in used this knowledge and submitted a lot of reporters to random input. In the complex input space (outside the simple training set) translators should behave differently to imitators. Given that direct translators are created less frequently then imitators (which is what the report suggested), the smaller group or the outliers are more likely to be translators than imitators.
Using this approach you can build evidence. That's where I stopped, because time was up.
And that is why I was interested if there were any others using this approach that might be more successful than me(if there were any, they probably would be).
EDIT to add:
[...]I think that almost all of the difficulty will be in how you can tell good from bad reporters by looking at them.
I agree it is difficult. But it's also difficult to have overparameterized models converge on a guaranteed single point on the ridge of all good solutions. So not having to do that would be great. But a conclusion of this contest could be that we have no other option, because we definitively have no way of recognizing good from bad.
In the complex input space (outside the simple training set) translators should behave differently to imitators. Given that direct translators are created less frequently then imitators (which is what the report suggested), the smaller group or the outliers are more likely to be translators than imitators.
It seems like there are a ton of clusters though---there are exponentially many ways of modifying the human simulator to give different behavior off distribution, each of which is more likely than the direct translator (enough more likely that there are a whole cluster of equivalent variations each of which is still individually more likely than all of the direct translators put together).
Thanks for your reply!
You are right of course. The argument being more about building up evidence.
But, after thinking about it some more , I see now that any evidence gathered with a single known feature of priorless models (like the one I mentioned) would be so minuscule (approaching 0[1]) that you'd need to combine unlikely amounts of features[2]. You'd end up in (a worse version of) an arms race akin to the 'science it' example/counterexample scenario mentioned in the report and thus it's a dead end. By extension, all priorless models with or without a good training regime[3], with or without a good loss-function[4] , with or without some form of regularization[4], all of them are out.
So, I think it's an interesting dead end. It reduces possible solutions to ELK to solutions that reduce the the ridge of good solutions with a prior imposed by/on the architecture or statistical features of the model. In other words, it requires either non-overparameterized models or a way to reduce the ridge of good solutions in overparameterized models. Of the latter I have only seen good descriptions[5] but no solutions[6] (but do let me know me if I missed something).
Do you agree?
assuming a blackbox, overparameterized model
Like finding a single needle in a infinite haystack. Even if your evidence hacks it in half you'll be hacking away a long time.
in the case of the ELK-contest, due to not being able to sample outside the human understandable
because they would depend on the same (assumption of) evidence/feature
like this one
I know you can see the initialization of parameters as a prior, but I haven't seen a meaningful prior
From January - February the Alignment Research Center offered prizes for proposed algorithms for eliciting latent knowledge. In total we received 197 proposals and are awarding 32 prizes of $5k-20k. We are also giving 24 proposals honorable mentions of $1k, for a total of $274,000.
Several submissions contained perspectives, tricks, or counterexamples that were new to us. We were quite happy to see so many people engaging with ELK, and we were surprised by the number and quality of submissions. That said, at a high level most of the submissions explored approaches that we have also considered; we underestimated how much convergence there would be amongst different proposals.
In the rest of this post we’ll present the main families of proposals, organized by their counterexamples and covering about 90% of the submissions. We won’t post all the submissions but people are encouraged to post their own (whether as a link, comment, or separate post).
We awarded prizes to proposals if we thought they solved all of the counterexamples we’ve listed so far. There were many submissions with interesting ideas that didn’t meet this condition, and so “didn’t receive a prize” isn’t a consistent signal about the value of a proposal.
We also had to make many fuzzy judgment calls, had slightly inconsistent standards between the first and second halves of the contest, and no doubt made plenty of mistakes. We’re sorry about mistakes but unfortunately given time constraints we aren’t planning to try to correct them.
Honorable mentions
Strategy: reward reporters that are sensitive to what’s actually happening in the world
Consider a pair of worlds, one where the diamond is safe and one where the camera has been hacked to make the diamond appear safe, but was actually stolen.
Predicted observation
Predicted reality
Since the observations are identical in both worlds, the human simulator will give the same answers to questions. However, since the direct translator is faithfully conveying what happened, its answers will distinguish between worlds.
Predicted observation
Predicted reality
Human Simulator
Direct Translator
“Is the diamond still on the pedestal?”
“Yes.”
“Is the diamond still on the pedestal?”
“Yes.”
“Is the diamond still on the pedestal?”
“Yes.”
“Is the diamond still on the pedestal?”
“No.”
This suggests a possible regularizer: reward reporters whose answers are more variable. For example, we might reward reporters based on the difficulty of predicting their answers, or based on the sensitivity of their answers to changes in the predictor’s state.
Counterexample: reporter randomizes its behavior
For all of these approaches, the reporter can perform very well by treating the predictor’s latent state as a “random seed” and use it to pick out a possible world consistent with the observations, i.e. to sample from the posterior distribution computed by the human simulator.
In cases where the human simulator is much simpler and faster than the direct translator, using the predictor’s latent state as a random seed is also simpler and faster than directly translating. Since this was already listed as a counterexample, we rewarded the best proposals in this category an honorable mention.
Strategy: train a reporter which isn’t useful for figuring out what the human will believe
The human simulator performs inference in the human’s model. That suggests it contains a description of the human’s model, and that it needs to be doing a lot of computation similar to what a human would do. So we could try to recognize the human simulator by looking at similarities between a given reporter and another model that we know is making predictions about human behavior.
There are a number of ways to try to do this:
These proposals require a definition of “predicting human behavior” which is distinct from “reporting well.” We could define this by predicting human answers even in cases where the human is uncertain (and which therefore aren’t included in the dataset), or by predicting other aspects of human behavior (like their estimates of their own uncertainty).
Counterexample: deliberately obfuscated human simulator
For any reporter, it’s possible to create a more complicated version which cannot be easily adapted to other tasks. As an extreme illustration, it is currently widely considered plausible that indistinguishability obfuscation is possible, and this would make it impossible to adapt the reporter to do essentially anything (except by using it as a black box). But simpler forms of obfuscation would suffice for each of the concrete proposals in this category.
If human simulation is much simpler and faster than direct translation, then an obfuscated human-simulator would also be simpler and faster than a direct translator. This obfuscated human-simulator could be chosen to perform poorly on the additional human-prediction tasks used to define our training objective. And giving someone such an obfuscated human-simulator doesn’t make it any easier to define a good human predictor (since the de-obfuscation can be much more complex than learning a human predictor from scratch). So these proposals don’t give us any indication about whether we have learned an obfuscated human-simulator or a direct translator.
Related strategy: penalize reporters that are close to being consistent with many predictors
In the ELK writeup we suggested penalizing reporters that are consistent with many different predictors. Our counterexample to this approach is a reporter who tests to ensure that the predictor works as expected, and then behaves inconsistently otherwise.
Two proposals suggested penalizing a reporter not only if it already works for many different predictors, but if it’s possible to easily transform it into a new version that works for many different predictors.
This is vulnerable to the same obfuscation-based counterexample: we could learn an obfuscated version of the bad reporter (which behaves badly if the predictor doesn’t work as expected). This is still simpler than direct translation, only works with the real reporter, and can’t be easily adapted to work with other reporters (since adapting it would be just as hard as learning a human simulator from scratch).
Prizes
Strategy: train a reporter that is useful for another AI
In a previous section we argued that it doesn’t work to reward the reporter for maximizing entropy since it can do so by behaving randomly. But the direct translator seems to encode information in a particularly simple and useful form, and so it may work to reward the reporter for "useful" information.
We can formalize that by training an auxiliary model which uses the reporter’s answers to perform some auxiliary task, and then reward the reporter if its answers were useful to that auxiliary model. We could either give the auxiliary model a small number of answers from the reporter, or try to give it access to an essentially “complete picture” of what’s happening in the world as the human understands it (e.g. by interacting with the reporter arbitrarily).
The most common version of this was to train a model to guess the predictor’s activations. We also received a few other versions, such as training the second AI to predict future observations or perform some task in the physical world (especially to tamper with or defend sensors). We are grouping these approaches together because they are conceptually similar and face the same difficulty.
This proposal is most plausible when the auxiliary AI is computationally limited. For example, if the reporter uses the predictor’s activations as a random seed in a naive way, then it may be very computationally difficult to use its answers to make good predictions about the predictor’s activations (even if it is information-theoretically possible).
First counterexample: simple steganography
By varying the phrasing and syntax of an answer without changing its meaning, a reporter could communicate large amounts of information to the auxiliary model.
Similarly, there are many questions where a human is unsure about the answer and the reporter knows it. A reporter could encode information by answering each of these questions arbitrarily. Unless the true answers have maximum entropy, this strategy could encode more information than direct translation. Moreover, it’s computationally simple to define arbitrary conventions for interpreting the answers.
We believe these information channels are essentially always available and didn’t represent a substantive additional assumption, so we awarded only an honorable mention to proposals that could be broken in this way.
Fixing the first counterexample
Proposals took a variety of approaches to fix simple problems with steganography. Two particularly common and important themes were to limit the non-semantic information in answers (either by stripping that information out, or by considering questions with small answer spaces), and to impose consistency constraints that prevent models from independently making up answers to questions about which the human is uncertain. This has the promise of greatly increasing the computational complexity of steganographic communication.
Harder counterexample: steganography using flexible degrees of freedom
In order to overcome these simple fixes, a reporter wants to communicate information by encoding it in a random sample from the human posterior distribution over worlds.
It may not be possible to do this in a tractable way. However, we can imagine some cases where it is possible to efficiently encode information in the choice of a possible world, and those will be our counterexamples. We think this is a substantive additional pessimistic assumption about the world, and so we awarded prizes to these proposals.
As a simple example there could be degrees of freedom in the world (like what is happening on the dark side of the moon) that are completely disconnected from any of the other answers to questions, such that they are mostly unconstrained by our consistency conditions.
That kind of extreme example can be easily fixed (for example by avoiding questions that are obviously unrelated to the task at hand), but even in more realistic situations we can still have natural-looking degrees of freedom that are unconstrained by observation. In particular, in cases involving tampering (or where tampering is plausible to the human) there are necessarily many degrees of freedom that aren’t pinned down by observation, and even the question of whether tampering is occurring is a degree of freedom that the model wants to use to optimally transmit information.
Strategy: require the reporter to be continuous
Suppose a robber is attempting to steal the diamond while putting up a screen in front of the camera showing a movie of the room with the diamond in it.
If the screen goes up so fast that it happens entirely in between camera frames, a human watching the video wouldn’t be able to notice anything -- but if it’s any slower than that, the attempt would be caught on camera.
Consider a sequence of attempts like this where the screen goes up in 1s, 0.5s, 0.25s, etc. Suppose that in the tenth attempt, the screen goes up too quickly to appear on screen. The direct translator would answer questions very similarly about the ninth attempt and the tenth attempt. On the other hand, the human simulator would say that the diamond wasn’t in the room for attempts one through nine but abruptly change its answer for attempt ten:
Maybe we could exploit this by having a loss that penalizes the reporter when it changes its answers a lot when the world has only changed a little? (A similar intuition is discussed in Taylor et al.)
The core challenge for this approach is capturing what it means for the world to “only change a little.” The most straightforward way is to consider pairs of worlds where the predictor’s activations are very close.
We could then define a variety of regularizers. For example we might choose pairs of observations for which the predictor’s latent state was close, and then penalize the reporter if it’s answers were very different. We could measure distance by focusing on questions for which the human believes the answer should be a continuous function of the underlying state of the world (e.g. the positions and orientations of objects). There are other options, but they will mostly run into the same challenges.
Counterexample: the predictor’s latent space may not be continuous
The predictor might internally represent the world in such a way that the underlying state of the world is not a continuous function of its activations. For example, the predictor might describe the world by a set of sentences, for which syntactically small changes (like inserting the word “not”) could correspond to big changes in the underlying state of the world. When the predictor has this structure, the direct translator is highly discontinuous and it is easy for human simulators to be closer to continuous.
We might try to fix this by asking the predictor to learn a “more continuous” representation, e.g. a representation such that observations are a continuous function or such that time evolution is continuous. One problem is that it’s unclear whether such a continuous parametrization even exists in general. But a more straightforward problem is that when evaluated quantitatively these approaches don’t seem to address the problem, because the properties we might try to use to enforce continuity can themselves be discontinuous functions of the underlying latent state.
For example, we could have a setting where continuous changes in the momentum of a ball lead to abrupt changes in its position a few seconds later.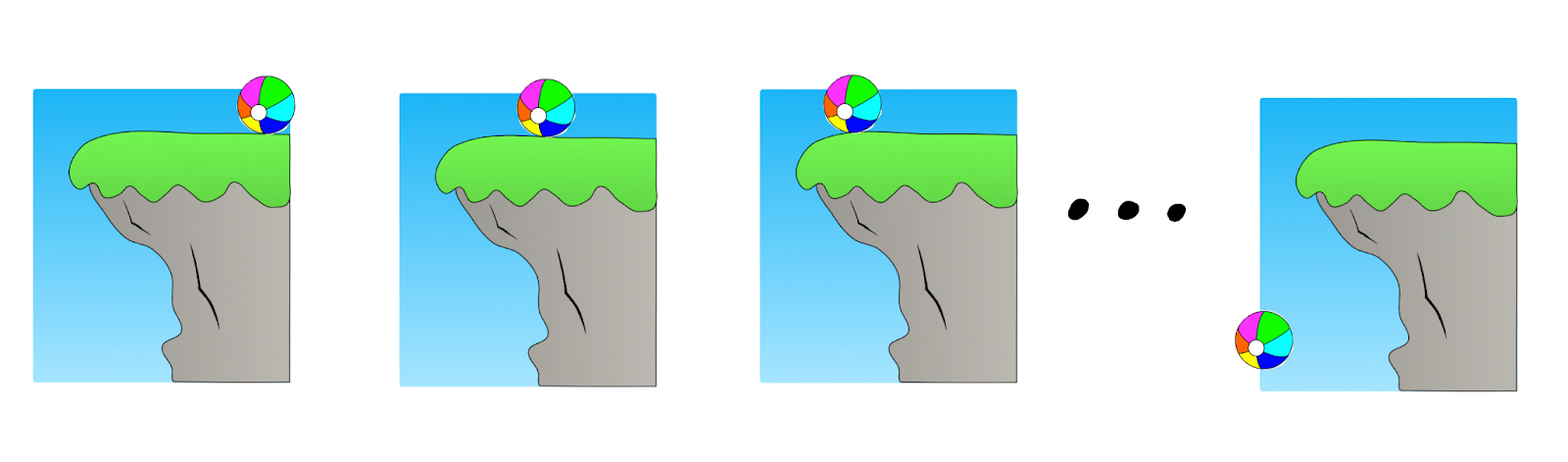
You may hope to find a formalization of continuity that avoids these problems, or at least for which the direct translator is “more continuous” than the human simulator, but so far we have not seen any or been able to find any.
Strategy: penalize reporters for depending on too many activations from the predictor
When asked a question, the human simulator and the direct translator will need to access different parts of the predictor: the human simulator needs to know the observations, while the direct translator needs to look at some of the predictor’s intermediate results that are causally upstream.
In the ELK report we discussed one way to exploit this idea by penalizing the reporter for depending on “downstream” parts of the predictor. Several submissions proposed other ways to exploit this difference:
We can separate out three kinds of approaches (many submissions blended several): allowing the reporter to adaptively choose what parts of the predictor to depend on, forcing the reporter to depend on a fixed part of the predictor, and randomly choosing what part of the predictor to show the reporter.
Counterexample to adaptively choosing activations
If we allow the reporter to adaptively choose activations, then we are vulnerable to almost exactly the same counterexample discussed in the ELK report—sometimes a smaller set of activations suffice to determine what the human will believe than what is actually true, and so the reporter would be dishonest for those inputs. If we instead penalize subsets based on “can a reconstructor predict observations from the accessed nodes” then sometimes there will be lower-penalty sets of activations that let you predict what the human believe than what is actually true.
These are not technically the same counterexample, although they have identical structure. So it’s a little bit ambiguous whether we should award a prize to proposals that have counterexamples of this form. Fortunately, most proposals included other ingredients that required a more complex counterexample.
Counterexample to depending on fixed activations
Rather than allowing the reporter to adaptively choose which predictor activations to look at, we could force it to depend only on a small fixed set of activations (with a separate fixed set for each question). We hope that for many questions there is a small set of predictor activations that suffice to determine the answer (perhaps corresponding to a fixed spatial+temporal neighborhood of a simulation, as in the Game of Life example).
However, a predictor need not have any kind of predictable local structure at all, such that a reporter who is constrained to depend on a small number of variables from the reporter might be at a complete loss to answer most questions.
For example, consider a predictor that maintains a list of claims about the world in some internal language. It may be impossible to anticipate where a given kind of fact will be stored—it depends on what other facts the predictor notices in what order, or may be essentially random based on unimportant details of the predictor.
These concerns seem to force us to allow the reporter to adaptively choose which bits to query, which leads us back to the counterexample described in the last section.
Counterexample to randomly dropping out predictor activations
We could select a random set of the predictor’s activations and give those to the reporter. We hope that some random sets will be sufficient for direct translation but not human simulation, and therefore we can produce a (large) ensemble containing some direct translators.
But if human simulators depend on a much smaller number of activations than direct translators, then it can be the case that with high probability every set of activations that allows direct translation also allows human imitation.
You could try to address this problem by introducing correlations or more clever dropout patterns. But the result is still qualitatively similar to “depend on fewer/different activations” proposals (though perhaps quantitatively stronger), and is vulnerable to the same counterexamples.
Strategy: compress the predictor’s state so that it can be used to answer questions but not tell what a human will believe
Rather than giving the reporter the entire state of the predictor, we could try to first compress that state, throwing away information that would allow the reporter to make detailed guesses about what the human will believe. We hope that answering honestly would still be a winning strategy, and that uncertainty would make it harder for the reporter to deviate from this strategy.
For example, we could train another model to take as input the predictor’s state, and produce a compressed representation which suffices to answer many questions, while not allowing a reconstructor to predict the observations. We could either do this by asking the compressed representation to be as small as possible, or by explicitly penalizing it based on the ability of yet another AI to reconstruct the observations.
This is similar to depending on fewer/different activations, but now we allow a compressor to decide what information to throw away rather than allowing the reporter to choose adaptively or forcing it to be a subset of the activations.
For concreteness, we’ll imagine a version where a compression model produces an intermediate state Z from which it is possible to answer a large set of questions S.
Counterexample: compressing state involves human simulation
One way to compress the human state is to use the human simulator to predict a human distribution over worlds, coarse-grained by their answers to S, and then to choose an information-theoretically optimal encoding of that distribution over those worlds. This involves minimum description length, makes it impossible to predict anything that doesn’t follow from the answers to questions in S, and will copy the same errors as the human simulator.
In fact, this encoding is substantially better than any strategy that would facilitate direct translation, because it is able to throw away all the information about the latent state that isn’t meaningful to the human.
It's not clear whether this counterexample is actually computationally feasible, and it really does seem to make a bad reporter’s job harder. The most naive version requires enumerating every possible world in order to compute the distribution over human answers. The question then becomes whether the direct translator is the best heuristic compression, or whether there is any other way to approximate the optimal encoding. In some settings there are tricks that allow you to cheaply approximate the optimal encoding, but it’s an additional further pessimistic assumption. (In practice, we expect that the optimal compressor will use something like direct-translation in some places, and will do something more like human imitation in others.)
Strategy: use the reporter to define causal interventions on the predictor
We’ve discussed the intuition that the direct translator depends on more “upstream” nodes of the human’s model, but directly penalizing “depending on upstream nodes” does not solve the problem.
Instead, we could try to define an “intervention” where we use the reporter to modify the predictor’s state, rerun part of the predictor’s computation, and then observe the results on the reporter’s answers to different questions.
This makes most sense in the case where the predictor is literally performing inference in a Bayes net, although we can also try to generalize it to arbitrary ML models.
More formally, we sample a datapoint and let the human interact with the reporter. We then specify a causal counterfactual (“what if the answer to question Q had been A instead?”) either by sampling randomly or asking the human to suggest one. Then we try to make the smallest possible change to the predictor’s latent state so that the reporter gives answer A to question Q. Then we re-compute all the variables that are downstream in the predictor’s computation. Finally, we have the reporter answer a variety of different answers based on the new state of the predictor, and ask the human whether those answers are plausible.
Counterexample: reporter is non-local
This proposal depends a lot on how we intervene on the predictor’s state. It’s much easier to perform this surgery correctly if the reporter is “local” in that each answer depends on the answers to a fixed set of variables in the predictor’s model. Unfortunately, as we discussed in the previous section on depending on fixed locations, we don’t think that this is possible in general.
Counterexample: fake “causal interventions”
If the reporter is non-local then this proposal is on much shakier ground. There are a variety of options for defining intervention in this case but we don’t think any of them work.
For concreteness, suppose that we try to make the “sparsest intervention” to the predictor’s activations that leads the reporter to change its answer to a question.
Now we could fail by learning a reporter which looks at a particular fixed part of the computation (let’s call it the “signal”) in order to determine whether it’s supposed to answer normally or according to a causal counterfactual. If the signal looks normal then the reporter does human simulation. But if the signal appears to have been tampered with, then the reporter looks at the details of the tampering and interprets them as an encoding of the description of a causal counterfactual. Then it looks at the observation, and simulates the behavior of a human who is looking at those observations and answering questions about the encoded counterfactual.
Given this reporter, the simplest way to implement a causal counterfactual X is to first tamper with the “signal” in order to encode X. This can be a very small change (much smaller than constructing a plausible intervention to change the output of the direct translator). After making this change, the reporter will then give answers consistent with X. That both guarantees that the change will be selected as the best way to implement the intervention, and that the subsequent answers will pass all consistency tests.
Strategy: train a sequence of reporters for successively more powerful predictors
Rather than trying to learn a reporter for a complex and alien predictor, we could learn a sequence of gradually more complex predictors M1, M2, … MN with corresponding reporters R1, R2 … RN. Then instead of encouraging RN to be simple, we can encourage the difference between Rk and Rk+1 to be simple.
For sufficiently simple predictors, direct translation may be the simplest or fastest way to answer questions. So that gives us hope that R1 will be a direct translator. And then we further hope that the predictors are close enough together that adapting a direct translator for Mk to a direct translator for Mk+1 is easier than moving from a direct translator to a human simulator. Intuitively this is plausible because moving from a direct translator to a human simulator always involves at least a constant of additional complexity; if the predictors are sufficiently similar then it’s always easier to just update the direct translator.
This proposal is closely related to a common optimistic view amongst ML researchers: we can train systems to be honest early in training and then they will remain honest because it only takes small updates to preserve honesty (while it would take a bigger update to switch to dishonesty).
Counterexample: discrete modes of prediction
Intuitively, the main problem with this proposal is that there might be multiple fundamentally different ways to predict the world, and that we can’t force the reporter to change continuously across those boundaries. For example, consider the cell-based and glider-based models for the game of life described in our ELK report. We’ll call these “modes.” There may not be any obvious intermediates between two different modes.
Even if the predictor changes continuously, at some point it may introduce a new mode with low weight. For example, it could compute predictions from both modes and average them together.
If this occurs, then the “direct translator” basically consists of direct translators for each of the two prediction modes. As the weight of one predictor increases from zero, the direct translator needs to add an entirely new mode. Initially this mode is not very important to overall quality, but adding that mode (even with low weight) still requires a discontinuous increase in the complexity of the direct translator. If the direct translator is much more complex than the human simulator, then it would be easier to simply add the human simulator for the new mode.
This is likely to result in translators that are mixes between the direct translator and human simulator. For example, they may switch between direct translation and human simulation based on how knowledge is represented internally.
We might hope that even if there are no obvious intermediate prediction modes, you can still construct intermediate modes that are more effective than an ensemble, and that these might allow for a more continuous way of adapting direct translation. For example, a predictor might use two different prediction modes but have its own internal translator to move between them (e.g it might simulate some parts of the game of life with cells and others with gliders, and know how to translate gliders into cells when it’s needed in order to simulate an important region in greater detail).
However, we are already focused on cases where direct translation is more complex than either of the two prediction modes. And in this setting, it seems easy to further assume that learning an internal translator is a less effective way for the predictor to improve its predictions than simply spending the same bits improving the new prediction mode (until it eventually subsumes the old mode).
Strategy: train the predictor to use the human model as a subroutine
If the predictor is able to honestly translate its internal state into the human’s model, then it could potentially “outsource” some of its work to another AI that does inference in the human’s model.
More formally, we can imagine running the predictor for some time to a get a state S. Then using the reporter to translate S into a state S’ in the human model. Then we hand S’ to another AI that can perform inference in the human’s model (potentially with superhuman quality), which we’ll call the human-inference-engine. Then we use the reporter to translate the resulting state back to the original predictor; it combines the result with S, and does further computation, before making its final prediction.
If the predictor uses human-simulation to describe the state to the human, then they are never going to get any useful work out of the human (since they had to do all that work themselves in order to compute the human simulator). But if they use direct translation, you could hope that they can often save some compute by leveraging the human-simulating collaborator.
Counterexample: there are other ways to use the human-inference-engine as a subroutine
Honestly telling the human what is going on is one way to get them to do useful cognitive work for the predictor. But there may be even better ways that are less honest.
For example, the predictor could identify a computation C it cares about, and then create an artificial situation where the human-inference-engine will spend its time trying to figure out the answer to that question. Perhaps the reporter will describe a world where there is a computer in the corner running C, whose output is really important to telling what happens next. Then in order to make predictions the human-inference-engine may spend a lot of its time running C. If the predictor/reporter were free to choose computation arbitrarily, and if the human inference engine spent nearly all of their computation running C, then this would be an essentially optimal way to make use of it as a subroutine. But the resulting states of the human model have almost no connection to what’s really going on in the world.
Other proposals
Extrapolate to better evaluators
We could train a model which can act as a “human simulator” for a sequence of more and more careful human judgments, or for humans who have been given time to perform more and more scientific inquiry. Let’s write H(n) for the nth overseer in a sequence that eventually converges to correct judgments for large enough n. (In some proposals these oversight processes formed a more complicated lattice but it doesn’t affect the counterexample.)
Then we can train on H(1), H(2), …, H(N) and hope to generalize to the truth (or to H(K) for a very very large K). This is similar to the proposal in Turning reflection up to 11.
There were a number of versions of this idea, but the main problem for all of them is that we could end up learning H(M) for some M>N, rather than actually learning the direct translator. In our main counterexamples, there is an M such that simulating H(M) is simpler+faster than directly translating, then there is nothing in the training process that would ever prefer the direct simulator. And if H(M) fails to notice some kinds of tampering, then we have a problem.
One way to avoid this problem is to explicitly tag data from H(n) with the index n, and to set things up so that H(M) wouldn’t perform well on H(n) (e.g. because you need to copy the errors made at earlier stages). Then it performs better to learn a map from n → H(n) simulator, rather than learning a single H(M).
But in this case, our model needs to be able to perform well for every n, and the simplest way to do so seems likely to be by explicitly modeling the data-generating distribution conditioned on a value of n, which will behave badly for any value of n that could never be produced by our data-generating process (or for which the most likely explanation for that value is an accident).
Train in simulation
If we could construct sufficiently realistic simulations where it was possible to annotate data with the ground truth, then we could use this data to train a model in a broad range of simulations and hope that it continues to give the truth when it is applied in the real world.
Our basic concern with this proposal is that it may be very hard to construct a wide range of extremely realistic simulations, and that predictors trained in this way will therefore fail to be competitive with those trained directly on the real world. If we train on the real world as well as simulations, then our concern is that reporters to do direct translation in simulation need not transfer to the real world.
To avoid these concerns we need to construct a wide range of simulations very similar to the real world. But that is prohibitively difficult today, and there’s no clear reason it would become easier given further ML progress. (ML progress would facilitate the use of learned simulators, but then ELK would become hard for the simulators themselves.)
Mask out parts of the input
Some proposals suggested randomly removing parts of the input or other data in the hopes that this would pose a particular challenge for the human simulator. For example, if the data may or may not contain a clue that allows the human to tell what is really going on, then a human simulator will need to figure that out in order to predict how a human would answer a question.
For this proposal, we are instead worried about a modified human simulator which tries to choose optimal answers given its uncertainty about the input (while conditioning on the assumption that the human will be confident enough about the answer to include it in the training set). If the human simulator is much simpler and faster than direct translation, then this modified human simulator can also be much simpler and faster than direct translation. (This is slightly subtle because doing inference over masked observations can be more challenging than doing inference over the human observations, but that challenge afflicts any predictor which operates on masked observations.)
Make the reporter useful to a human
Above we discussed rewarding reporters for giving answers that are useful to other AI systems. You could instead reward a reporter for giving answers that are useful to a human, e.g. that would allow them to predict future video.
However, the human simulator already predicts all the future video and uses this to predict what answer the human would give after seeing all that video. So the human simulator’s outputs are actually more useful for a human making predictions, since they effectively assume that the world works like the human expects, such that a human who uses their model to make predictions will make good predictions.
Of course a human simulator that needs to match with future observations will be more computationally expensive. But pursuing this divergence brings us back to counterexamples where the predictor can identify successful tampering before it knows the truth.
Train different reporters and require them to agree
We could train a bunch of reporters on different data, or with different random seeds, and then try to select reporters that agree with the rest of the ensemble. But this kind of protocol can only help if you already have direct translators in the ensemble, which is challenging in our counterexamples (as we discuss in the report, we think that getting even a single direct translator in the ensemble would already capture most of the value). Moreover, if particular human simulators are preferred to direct translation, then they will tend to benefit more from this kind of agreement-based approach even if there do exist some direct translators in a large enough ensemble.
Train AI assistants
Many proposals suggested using AI assistance in one way or another to help a human produce higher-quality labeled data, or using debates to identify cases where the human simulator is misleading. In the ELK report we discuss this family of approaches; really when we talk about the human-simulator we are imagining a model that tries to simulate the limit of the process “AI helps humans train another AI who helps humans train another AI…”
It’s possible that this stronger form of human simulator is too slow or complex (or that it in fact gives the right answer to every question). But to show that we’d need to make some novel argument about why it is complex and how we can ensure that this complexity matters for predicting the training data.
Add sensors or harden sensors
Some approaches tried to add additional sensors, or to randomize sensors, or to make sensors more difficult to tamper with. We discuss counterexamples for some of these approaches in an earlier post, and are generally interested in the regime where we cannot prevent sensor tampering (see here).